|
ACCESS THE FULL ARTICLE
No SPIE Account? Create one
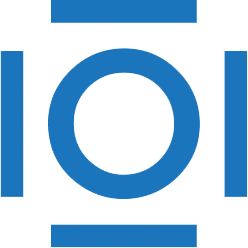
CITATIONS
Cited by 5 scholarly publications.
Neural networks
Numerical integration
Computer simulations
Neurons
Civil engineering
Numerical simulations
Damage detection