|
ACCESS THE FULL ARTICLE
No SPIE Account? Create one
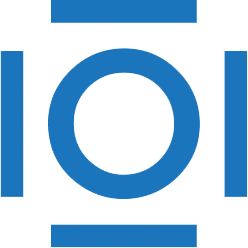
CITATIONS
Cited by 8 scholarly publications.
Composites
Synthetic aperture radar
Image classification
Earth observing sensors
Landsat
Remote sensing
Feature extraction