|
ACCESS THE FULL ARTICLE
No SPIE Account? Create one
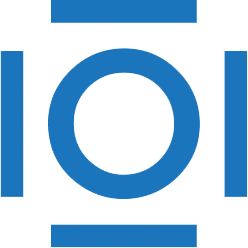
CITATIONS
Cited by 1 scholarly publication.
Control systems
Classification systems
Chemical elements
Defense and security
Sensors
Target detection
Telecommunications