|
ACCESS THE FULL ARTICLE
No SPIE Account? Create one
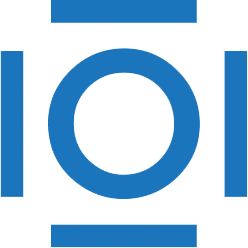
CITATIONS
Cited by 3 scholarly publications.
Wavelets
Reflectivity
Absorbance
Hyperspectral imaging
Short wave infrared radiation
Mahalanobis distance
Absorption