|
ACCESS THE FULL ARTICLE
No SPIE Account? Create one
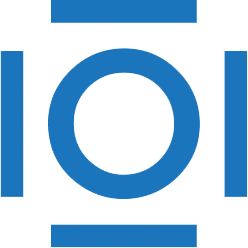
CITATIONS
Cited by 10 scholarly publications.
Neural networks
Optical proximity correction
Image classification
Lithography
Feature extraction
Machine learning
Optical lithography