|
ACCESS THE FULL ARTICLE
No SPIE Account? Create one
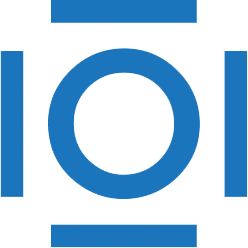
CITATIONS
Cited by 5 scholarly publications.
Filtering (signal processing)
Particle filters
Particles
Time metrology
Nonlinear filtering
Electronic filtering
Systems modeling