|
ACCESS THE FULL ARTICLE
No SPIE Account? Create one
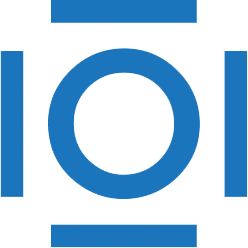
CITATIONS
Cited by 1 scholarly publication.
Hyperspectral imaging
Near infrared
Principal component analysis
Raw materials
Cameras
Imaging spectroscopy
Spectrographs