|
ACCESS THE FULL ARTICLE
No SPIE Account? Create one
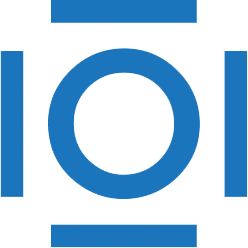
CITATIONS
Cited by 26 scholarly publications.
Mahalanobis distance
Hyperspectral imaging
Statistical analysis
Silicon
Sensors
Detection and tracking algorithms
Defense and security