|
ACCESS THE FULL ARTICLE
No SPIE Account? Create one
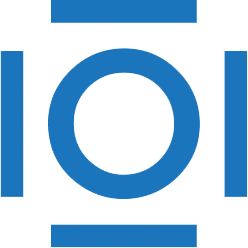
CITATIONS
Cited by 1 scholarly publication.
Motion models
Image filtering
Image processing
Detection and tracking algorithms
Convolution
Motion estimation
Evolutionary algorithms