|
ACCESS THE FULL ARTICLE
No SPIE Account? Create one
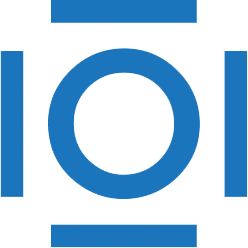
CITATIONS
Cited by 40 scholarly publications.
Particles
Particle filters
Nonlinear filtering
Monte Carlo methods
Signal to noise ratio
Algorithms
Error analysis