|
ACCESS THE FULL ARTICLE
No SPIE Account? Create one
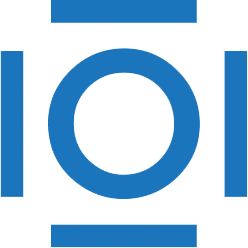
CITATIONS
Cited by 2 scholarly publications.
Calibration
Error analysis
Monte Carlo methods
Principal component analysis
Data modeling
Sensors
Statistical analysis