|
ACCESS THE FULL ARTICLE
No SPIE Account? Create one
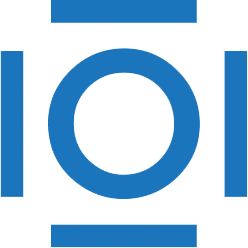
CITATIONS
Cited by 18 scholarly publications.
Principal component analysis
Deconvolution
Turbulence
Image quality
Point spread functions
Image restoration
Expectation maximization algorithms