|
ACCESS THE FULL ARTICLE
No SPIE Account? Create one
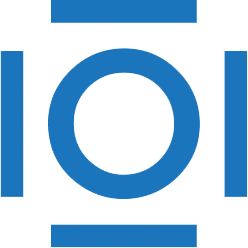
CITATIONS
Cited by 1 scholarly publication.
Data modeling
Quantization
Computer simulations
Data mining
Error analysis
Algorithm development
Mining