|
ACCESS THE FULL ARTICLE
No SPIE Account? Create one
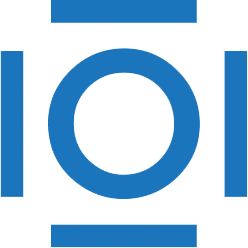
CITATIONS
Cited by 4 scholarly publications.
Autoregressive models
Structural health monitoring
Distance measurement
Principal component analysis
Pattern recognition
Mahalanobis distance
Performance modeling