|
ACCESS THE FULL ARTICLE
No SPIE Account? Create one
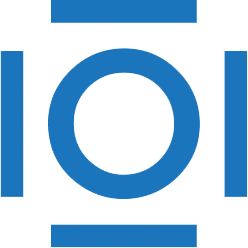
CITATIONS
Cited by 7 scholarly publications.
Reflectivity
Data modeling
Error analysis
Sensors
Optimization (mathematics)
Adaptive optics
Atmospheric modeling