|
ACCESS THE FULL ARTICLE
No SPIE Account? Create one
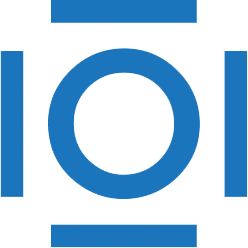
CITATIONS
Cited by 12 scholarly publications.
Hyperspectral imaging
Binary data
Chemical analysis
Matrices
Data centers
FT-IR spectroscopy
Image processing