|
ACCESS THE FULL ARTICLE
No SPIE Account? Create one
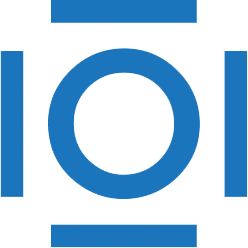
CITATIONS
Cited by 9 scholarly publications.
Genetic algorithms
Lead
Metals
Remote sensing
Soil science
Soil contamination
Data modeling