|
ACCESS THE FULL ARTICLE
No SPIE Account? Create one
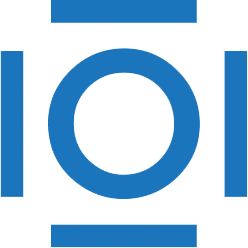
CITATIONS
Cited by 2 scholarly publications.
Reconstruction algorithms
Hyperspectral imaging
Optimization (mathematics)
Image compression
Compressed sensing
Convex optimization
Image restoration