|
ACCESS THE FULL ARTICLE
No SPIE Account? Create one
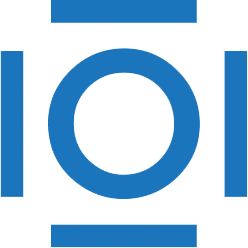
CITATIONS
Cited by 9 scholarly publications.
Brain-machine interfaces
Electroencephalography
Brain
Electrodes
Eye
Autoregressive models
Feature extraction