|
ACCESS THE FULL ARTICLE
No SPIE Account? Create one
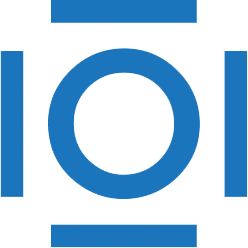
CITATIONS
Cited by 1 scholarly publication.
Computer simulations
Temperature metrology
Data modeling
Diagnostics
Liquids
Lithium
MATLAB