|
ACCESS THE FULL ARTICLE
No SPIE Account? Create one
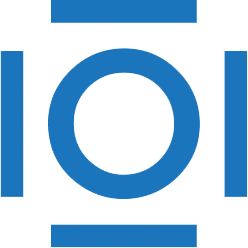
CITATIONS
Cited by 10 scholarly publications.
Explosives
Image filtering
Explosives detection
Matrices
Imaging systems
Binary data
Ground penetrating radar