|
ACCESS THE FULL ARTICLE
No SPIE Account? Create one
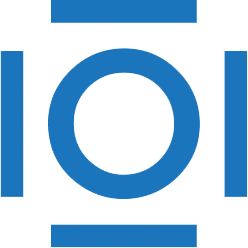
CITATIONS
Cited by 1 scholarly publication.
Data modeling
Data integration
Information fusion
Associative arrays
Data conversion
Sensors
Data fusion