|
ACCESS THE FULL ARTICLE
No SPIE Account? Create one
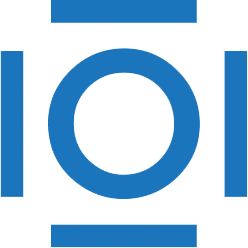
CITATIONS
Cited by 1 scholarly publication.
Data modeling
Fractal analysis
Prototyping
Binary data
Statistical analysis
Platinum
Brain