|
ACCESS THE FULL ARTICLE
No SPIE Account? Create one
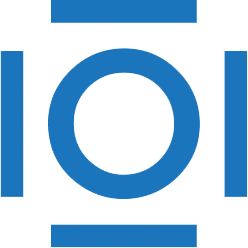
CITATIONS
Cited by 2 scholarly publications.
Binary data
Image filtering
Machine vision
Robots
Computer vision technology
Robot vision
Filtering (signal processing)