|
ACCESS THE FULL ARTICLE
No SPIE Account? Create one
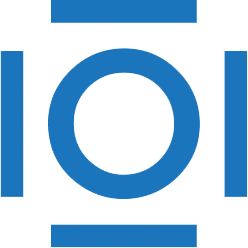
CITATIONS
Cited by 4 scholarly publications.
Image classification
Image segmentation
Machine learning
Sensors
Spatial resolution
Image analysis
Image processing algorithms and systems