|
ACCESS THE FULL ARTICLE
No SPIE Account? Create one
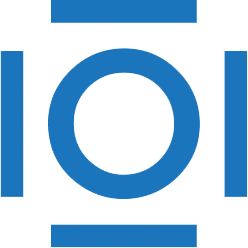
CITATIONS
Cited by 5 scholarly publications.
Bridges
Sensors
Associative arrays
Neodymium
Convex optimization
Data acquisition
Structural health monitoring