|
ACCESS THE FULL ARTICLE
No SPIE Account? Create one
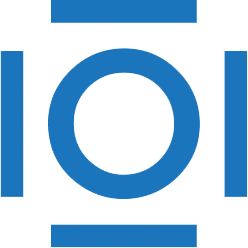
CITATIONS
Cited by 1 scholarly publication.
Machine learning
Statistical analysis
Statistical inference
Data modeling
Error analysis
Probability theory
Electrical engineering