|
ACCESS THE FULL ARTICLE
No SPIE Account? Create one
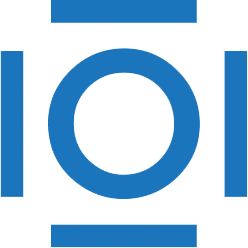
CITATIONS
Cited by 11 scholarly publications.
Visualization
Tumor growth modeling
Associative arrays
Independent component analysis
Visual process modeling
Tissues
Data modeling