|
ACCESS THE FULL ARTICLE
No SPIE Account? Create one
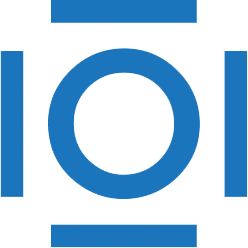
CITATIONS
Cited by 18 scholarly publications.
Image segmentation
Electroluminescent displays
Expectation maximization algorithms
Optimization (mathematics)
Hyperspectral imaging
Medical imaging
Image processing algorithms and systems