|
ACCESS THE FULL ARTICLE
No SPIE Account? Create one
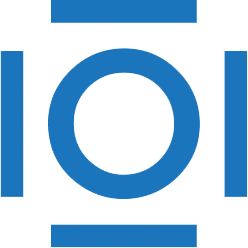
CITATIONS
Cited by 7 scholarly publications.
Liver
Image classification
Computed tomography
Binary data
Feature extraction
Classification systems
Computer aided diagnosis and therapy