|
ACCESS THE FULL ARTICLE
No SPIE Account? Create one
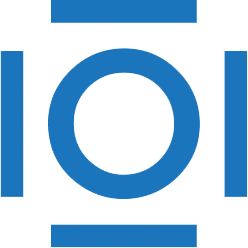
CITATIONS
Cited by 2 scholarly publications.
Buildings
Detection and tracking algorithms
Sensors
Visualization
Statistical analysis
Atmospheric modeling
Error analysis