|
ACCESS THE FULL ARTICLE
No SPIE Account? Create one
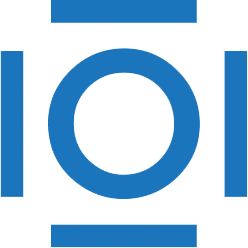
CITATIONS
Cited by 1 scholarly publication.
Image restoration
Point spread functions
Image processing
Deconvolution
Lawrencium
Expectation maximization algorithms
Cameras