|
ACCESS THE FULL ARTICLE
No SPIE Account? Create one
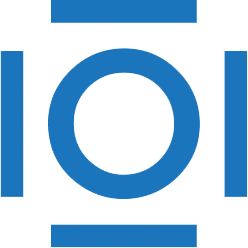
CITATIONS
Cited by 2 scholarly publications.
Associative arrays
Chemical species
Data modeling
Image storage
Image processing
Machine vision
Computer vision technology