|
ACCESS THE FULL ARTICLE
No SPIE Account? Create one
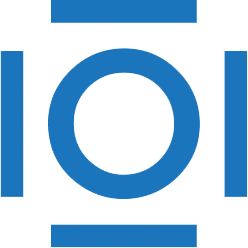
CITATIONS
Cited by 1 scholarly publication.
Data modeling
Hyperspectral imaging
Algorithm development
Electroluminescence
Process modeling
Diffusion
RGB color model