|
ACCESS THE FULL ARTICLE
No SPIE Account? Create one
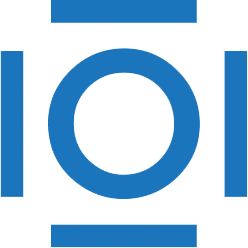
CITATIONS
Cited by 2 scholarly publications.
Data modeling
Sensors
Statistical modeling
Performance modeling
Statistical analysis
Data fusion
Data analysis