|
ACCESS THE FULL ARTICLE
No SPIE Account? Create one
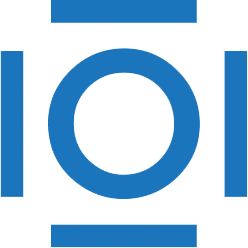
CITATIONS
Cited by 7 scholarly publications and 2 patents.
General packet radio service
Detection and tracking algorithms
Feature extraction
Sensors
Target detection
Explosives
Computing systems