Motivated by the potential of nondiffraction limited, real-time computational image sharpening with neural networks in astronomical telescopes, we studied wavefront sensing with convolutional neural networks based on a pair of in-focus and out-of-focus point spread functions. By simulation, we generated a large dataset for training and validation of neural networks and trained several networks to estimate Zernike polynomial approximations for the incoming wavefront. We included the effect of noise, guide star magnitude, blurring by wide-band imaging, and bit depth. We conclude that the “ResNet” works well for our purpose, with a wavefront RMS error of 130 nm for r0 = 0.3 m, guide star magnitudes 4 to 8, and inference time of 8 ms. It can also be applied for closed-loop operation in an adaptive optics system. We also studied the possible use of a Kalman filter or a recurrent neural network and found that they were not beneficial to the performance of our wavefront sensor. |
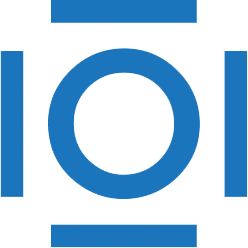
CITATIONS
Cited by 12 scholarly publications.
Neural networks
Point spread functions
Education and training
Wavefront sensors
Wavefronts
Stars
Astronomy