Building footprint segmentation from satellite and aerial images is an essential and challenging step for high-resolution building map generation. In urban management applications, such as building monitoring, infrastructure development, smart three-dimensional cities, and building change detection, building footprints are required to generate precise multiscale building maps. An efficient deep learning-based segmentation approach is proposed for multiscale building footprint extraction, and the results are presented for the most important challenges in photogrammetry and remote sensing, including shadows and occluded areas, vegetation covers, complex roofs, dense building areas, oblique images, and the generalization capability in different locations. The proposed method includes new dilated convolutional blocks containing kernels with different sizes to learn spectral–spatial relationships in multiscale satellite and aerial images with a high level of abstraction. The quantitative assessments of multiscale images from different locations with different spatial resolutions and spectral details show that the average F1 score and the average intersection over union for extracted footprints are about 86% and 76%, respectively. Compared with the state-of-the-art approaches, the proposed method has outstanding generalization capability and provides better performance for building footprint segmentation from multisensor single images. |
ACCESS THE FULL ARTICLE
No SPIE Account? Create one
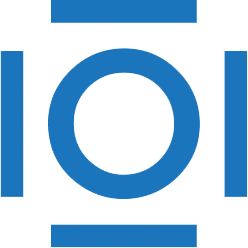
CITATIONS
Cited by 30 scholarly publications.
Image segmentation
RGB color model
Satellites
Earth observing sensors
Remote sensing
Satellite imaging
Convolution