|
ACCESS THE FULL ARTICLE
No SPIE Account? Create one
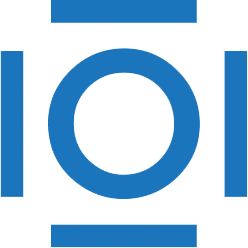
CITATIONS
Cited by 1 scholarly publication.
LIDAR
Neural networks
Machine learning
Inspection
MATLAB
Polarization
Optical inspection