One of the greatest challenges in many machine learning applications is to build robust classification models that reduce calibration efforts, ensure model transferability to unseen data distributions, and handle high semantic resolution datasets with a relatively large number of target classes. We present an operational domain adaptation framework for crop type verification from remote sensing data for integrated administration and control and farm management systems. It is part of the ag|knowledge crop monitoring platform and demonstrates a more robust spatiotemporal model transferability than conventional supervised crop type classification approaches, which use multitemporal and multispectral features to classify the specific characteristics of crop phenology patterns. The proposed method is based on a machine learning model that has been trained on particular similarity metrics between all target crop types. The metrics are derived by quantifying the similarity of vegetation index time series between the observed parcel and the aggregated time series of reference objects for each parcel and crop type. This method achieves higher classification results than a model trained on the pure remote sensing time series data, as the algorithm does not learn the temporal vegetation index patterns, but instead, the adversarial characteristics and the differences between each crop type. Moreover, it also reduces dimensionality as the time series are summarized through the respective similarity metrics. We compare both approaches through different classification algorithms. Overall, the achieved classification accuracy for more than 67 crop types scores more than 80%. |
ACCESS THE FULL ARTICLE
No SPIE Account? Create one
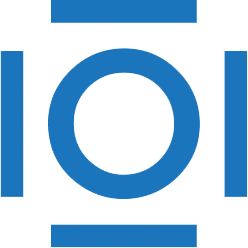
CITATIONS
Cited by 4 scholarly publications.
Data modeling
Remote sensing
Classification systems
Agriculture
Image classification
Machine learning
Systems modeling