Convincing depth estimation from monocular aerial images is a fundamental task in environment perception. We propose an efficient multilevel feature fusion network (MLFFNet) for estimating depths from aerial images. Specifically, the proposed MLFFNet consists of a multi-level attention pyramid (MAP) module and an adaptive feature fusion (AFF) module. The MAP module extracts the low-level and high-level useful information from the perspective of nonlocal spatial attention and channel attention, while the AFF module adaptively integrates the extracted multilevel features to enhance the estimated effect. Moreover, since images taken by drones have a large depth range, we designed a loss function suitable for aerial images. The evaluation experiments are performed on the MidAir dataset. Experimental results denote that our MLFFNet outperforms other depth estimation methods in predicting the depths. We also test several images from real-life scenarios, and our method can obtain reasonable outputs. |
ACCESS THE FULL ARTICLE
No SPIE Account? Create one
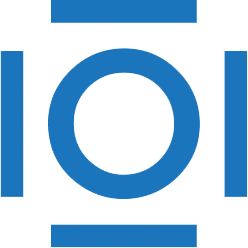
CITATIONS
Cited by 3 scholarly publications.
Image fusion
Lithium
3D modeling
RGB color model
Unmanned aerial vehicles
Image resolution
Cameras