Optical remote sensing images play an important role in many fields due to their natural visual representations. However, clouds obstruct a large proportion of recorded spectral signals and greatly affect the availability of high-quality optical images. In addition, the progress of cloud removal methods has long been restricted by the deficiency in theoretical basis and low computing power. With the advent of deep learning technology, this problem can be well addressed. We propose a network based on style transfer theory to remove thin and thick clouds in optical remote sensing images. An input data concatenation procedure with synthetic aperture radar (SAR) images is used to provide auxiliary information for the recovery of missing data caused by clouds. Moreover, the spatial attention mechanism adept at generating saliency information of image objects is also used to locate the clouds and corresponding shadows to make the network concentrate on the cloud region reconstruction task. Furthermore, to make the spatial attention mechanism self-adapted to the practical characteristics of clouded images, a loss function including a cloud mask is designed to assist the training step of the network. The proposed network is trained with a global open-source dataset and a region-specified dataset. The results of ablation experiments and comparison with baseline methods demonstrate that a high accuracy is guaranteed by the proposed model, even under high cloud coverage conditions [mean absolute error (MAE) = 0.0883, root-mean-square error (RMSE) = 0.1775, peak signal-to-noise ratio (PSNR) = 23.8, and structural similarity (SSIM) = 0.742). Using optical images independently to remove clouds results in poor results (MAE = 0.1156, RMSE = 0.2825, PSNR = 21.6, and SSIM = 0.596), which need to be addressed with data concatenation, such as SAR context information. Spatial attention mechanism plays an important role in improving the accuracy of cloud removal (MAE = 0.0251, RMSE = 0.0363, PSNR = 27.1, and SSIM = 0.876). For the missing values of optical images caused by cloud coverage, the Spatial-attention-CycleGAN proposed provides a potential solution for information recovery. |
ACCESS THE FULL ARTICLE
No SPIE Account? Create one
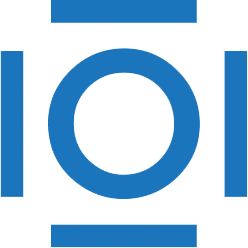
CITATIONS
Cited by 7 scholarly publications.
Clouds
Remote sensing
Synthetic aperture radar
Data modeling
Visualization
Gallium nitride
Reflectivity