Measurement of forest biomass is a time-, money-, and labor-consuming activity. Developing methodological approaches for quantifying biomass in natural ecosystems has motivated countless investigations in several vegetation types worldwide. In this study, we developed a model to estimate the above-ground biomass (AGB) in a study site spatially located in Western Pará state, Brazilian Amazon. We applied artificial neural networks and remotely sensed data for adjusting our model based on forest inventory data. We tested four vegetation indices retrieved from a Landsat-5 thematic mapper scene and assessed their correlations with the field measured AGB. We trained 286 artificial neural networks using the vegetation stratus (two phytophysiognomies: dense lowland ombrophilous forest and dense submontane ombrophilous forest) and the normalized difference vegetation index (NDVI) and aerosol-free vegetation index as predictors variables of the AGB. We selected the artificial neural network model showing higher correlation coefficient and concentration of residuals in the center classes, which indicates higher predictive capabilities of biomass estimation. The generated model architecture (4-13-1) was composed by 4 predictor neurons in the input layer, 13 neurons in the hidden layer, activated by logistic functions, and a single neuron (the AGB in ton · ha − 1) in the output layer, activated by an identity function. We observed that the NDVI and aerosol free vegetation index showed the best performance to estimate the AGB in the study area. Using artificial neural network and a Landsat-5 image combined, we were able to accurately predict (estimation error of ∼20 % ) AGB in tropical forest. This is a promising methodological approach that can be applied to assess ecosystem services related to carbon stock in tropical regions. |
ACCESS THE FULL ARTICLE
No SPIE Account? Create one
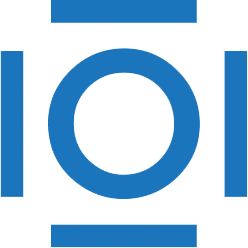
CITATIONS
Cited by 1 scholarly publication.
Vegetation
Artificial neural networks
Biomass
Biological research
Data modeling
Education and training
Atmospheric modeling