Ground point identification and segmentation are fundamental to the light detection and ranging (LiDAR) based environment perception because they affect the accuracy and computational efficiency in the following data processing steps. A common problem that results in over- and under-segmentation occurs when the objects of interest are nonhomogeneous, and the sampling density is uneven. This study addresses this issue using grid- and homogeneity-based approaches. This work began with a combined conditional and voxel filtering approach to shrink the spatial range and reduce the amount of point-cloud data. The spatial range was then divided into a concentric circular grid to reduce the complexity of data processing. A dynamic threshold model was used to classify the cloud points to improve the accuracy of ground-point identification on uneven, broken, and sloped roads. Additionally, a point cloud homogeneity model was used to optimize the ground point identification results in areas with vegetation. The experimental study was conducted based on the data provided in the semantic KITTI dataset, wherein comprehensive comparisons were made with state-of-the-art algorithms. The average precision, recall, F1, and running time of the proposed method were 92.5%, 90.89%, 0.92, and 0.146 s, respectively, outperforming most of the selected models in balanced accuracy and computational efficiency. |
ACCESS THE FULL ARTICLE
No SPIE Account? Create one
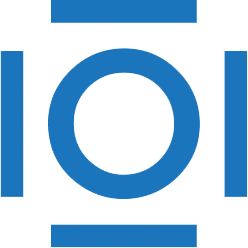
CITATIONS
Cited by 1 scholarly publication.
Point clouds
LIDAR
Vegetation
Tunable filters
Voxels
Semantics
Image segmentation