Self-supervised learning has been widely applied in the field of remote sensing image change detection (CD). Traditional self-supervised learning approaches, on the other hand, are limited to image-level classification tasks, do not provide pixel-level feature learning, and require a certain amount of labeled data for fine-tuning. We offer a self-supervised change detection method that does not require fine-tuning and allows for the acquisition of change result images without the use of labeled data. The suggested method is built on a contrastive learning framework that employs an UNet network to facilitate pixel-level feature reconstruction by introducing guided filtering, resulting in superior edge fit detection results than prior pixel-level self-supervised CD methods. Furthermore, the technique increases the accuracy of CD by incorporating a global contrast module. Simulation experiments were conducted on three public datasets, the Onera Satellite Change Detection dataset, the GF-2 Satellite Change Detection dataset, and the Jiangsu dataset, and compared with state-of-the-art CD methods. The result shows the effectiveness of the proposed algorithms based on evaluation metrics, such as overall accuracy, kappa coefficient, and |
ACCESS THE FULL ARTICLE
No SPIE Account? Create one
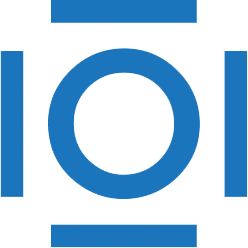
CITATIONS
Cited by 2 scholarly publications.
Tunable filters
Image filtering
Remote sensing
Image restoration
Feature extraction
Education and training
Image enhancement