Rice is the most important food crop in Taiwan. Early information on rice-growing conditions is thus vital for estimating rice production to guarantee national food security and grain exports. The rice-harvested area is conventionally inspected twice a year by costly interpretation of aerial photographs and intensive labor-field surveys. However, such methods of rice monitoring are inadequate for providing the government with timely information on rice-cultivated conditions. This study aims to use time series of Sentinel-1 synthetic aperture radar and Sentinel-2 multispectral data to develop a machine-learning approach for the early prediction of rice-growing fields in Taiwan. An object-based random forest (OBRF) was developed to process remotely sensed data for rice-cropping seasons from 2018 to 2021. The prediction results compared with the reference data showed that rice-growing fields could be accurately predicted before harvest, about three months for the first crop and two months for the second crop. The F-score and Kappa coefficient values achieved for the first crop were 0.87 and 0.85, and those for the second crop were 0.72 and 0.71, respectively. These findings were reaffirmed by close agreement between the official statistics and the rice area estimated from the satellite data, with the correlation coefficient of determination ( |
ACCESS THE FULL ARTICLE
No SPIE Account? Create one
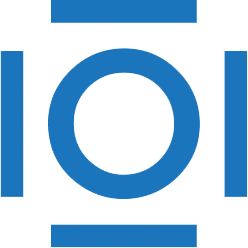
CITATIONS
Cited by 1 scholarly publication.
Satellites
Image segmentation
Data modeling
Machine learning
Satellite imaging
Synthetic aperture radar
Education and training