The generation of synthetic multispectral satellite images has not yet reached the quality level achievable in other domains, such as the generation and manipulation of face images. Part of the difficulty stems from the need to generate consistent data across the entire electromagnetic spectrum covered by such images at radiometric resolutions higher than those typically used in multimedia applications. The different spatial resolution of image bands corresponding to different wavelengths poses additional problems, whose main effect is a lack of spatial details in the synthetic images with respect to the original ones. We propose two generative adversarial networks-based architectures explicitly thought to generate synthetic satellite imagery by applying style transfer to 13-band Sentinel-2 level1-C images. To avoid losing the finer spatial details and improve the sharpness of the generated images, we introduce a pansharpening-like approach, whereby the spatial structures of the input image are transferred to the style-transferred images without introducing visible artifacts. The results we got by applying the proposed architectures to transform barren images into vegetation images and vice versa and to transform summer (res. winter) images into winter (res. summer) images, which confirm the validity of the proposed solution. |
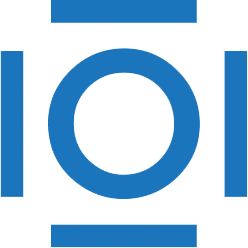
CITATIONS
Cited by 1 scholarly publication.
Image sharpness
Vegetation
RGB color model
Education and training
Gallium nitride
Land cover
Image quality