|
ACCESS THE FULL ARTICLE
No SPIE Account? Create one
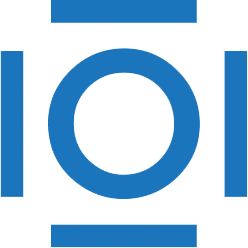
CITATIONS
Cited by 4 scholarly publications and 1 patent.
Image segmentation
Wavelets
Beam propagation method
Image processing algorithms and systems
Image quality
Image processing
Image classification