|
ACCESS THE FULL ARTICLE
No SPIE Account? Create one
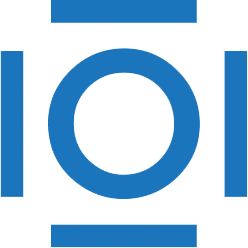
CITATIONS
Cited by 30 scholarly publications.
Synthetic aperture radar
Target recognition
Automatic target recognition
Convolutional neural networks
Signal to noise ratio
System on a chip
Feature extraction