|
ACCESS THE FULL ARTICLE
No SPIE Account? Create one
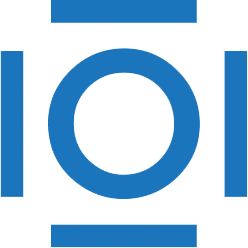
CITATIONS
Cited by 3 scholarly publications.
Databases
Performance modeling
Image quality
Data modeling
Feature selection
Image filtering
Feature extraction